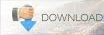

To make sensitive patient data available to others, data owners typically de-identify or anonymize the data in a number of ways, including removing identifiable features (e.g., names and addresses), perturbing them (e.g., adding noise to birth dates), or grouping variables into broader categories to ensure more than one individual in each category. This can severely delay the pace of research and, consequently, its translational benefits to patient care. Even when it is possible for a researcher to gain access to such data, ensuring proper data usage and protection is a lengthy process with strict legal requirements. While such datasets are potentially highly valuable resources for scientists, they are generally not accessible to the broader research community due to patient privacy concerns. Increasingly, large amounts and types of patient data are being electronically collected by healthcare providers, governments, and private industry. We discuss the trade-offs of the different methods and metrics, providing guidance on considerations for the generation and usage of medical synthetic data. Specifically, our cohort consists of breast, respiratory, and non-solid cancer cases diagnosed between 20, which includes over 360,000 individual cases. While the results and discussions are broadly applicable to medical data, for demonstration purposes we generate synthetic datasets for cancer based on the publicly available cancer registry data from the Surveillance Epidemiology and End Results (SEER) program. Metrics for evaluating the quality of the generated synthetic datasets are presented and discussed. In this paper, we evaluate three classes of synthetic data generation approaches probabilistic models, classification-based imputation models, and generative adversarial neural networks. These characteristics pose multiple modeling challenges. By and large, medical data is high dimensional and often categorical.
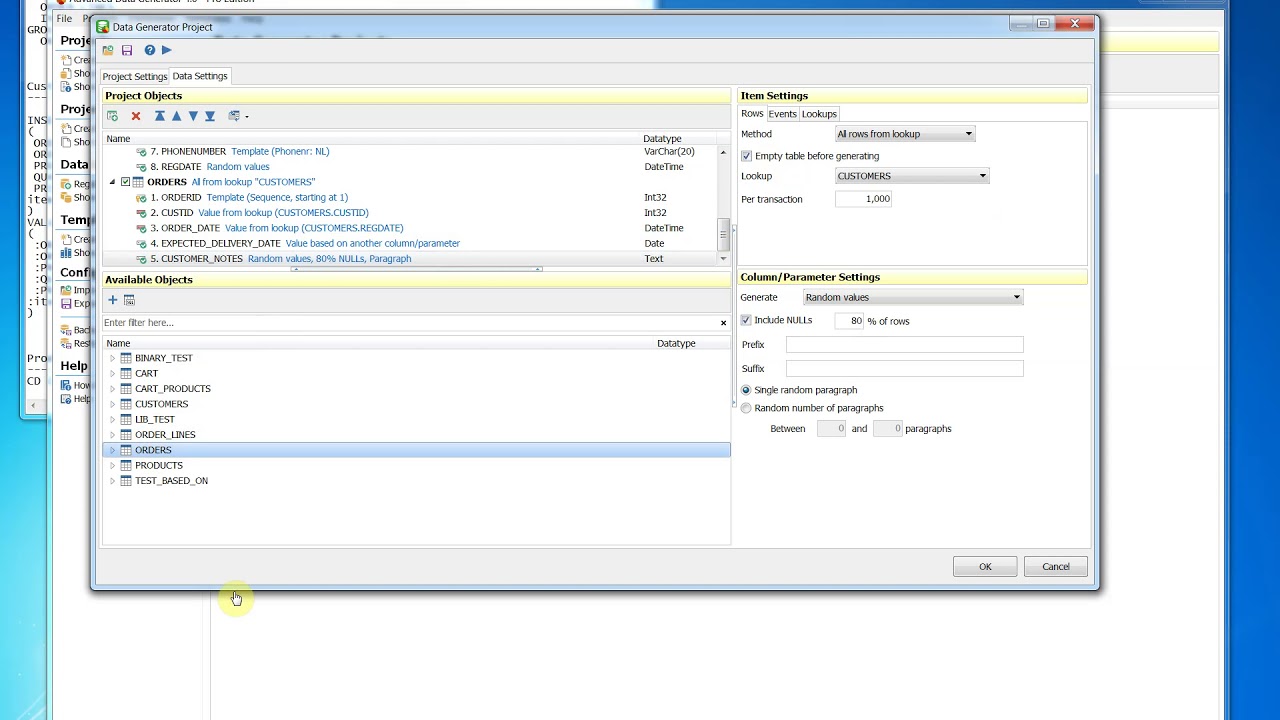
High-quality, realistic, synthetic datasets can be leveraged to accelerate methodological developments in medicine. A major reason for this has been the lack of availability of patient data to the broader ML research community, in large part due to patient privacy protection concerns. Machine learning (ML) has made a significant impact in medicine and cancer research however, its impact in these areas has been undeniably slower and more limited than in other application domains.
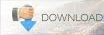